INTRODUCTION
Tobacco is the leading cause of preventable mortality1-3 and one of the biggest threats to public health. It kills at least 8 million people a year, of which more than 7 million are direct consumers and around 1.2 million are non-smokers exposed to the smoke of others. Unfortunately, reducing smoking consumption is not enough. In those smokers who reduce their consumption by more than 50%, the risk of mortality from all causes is similar to those who continue smoking4. In addition, despite worldwide awareness campaigns about the risks of smoking, not many people understand the specific health risks of inhalation. For example, a study conducted in China in 2009 revealed that only 38% of smokers knew that tobacco causes coronary heart disease, and only 27% knew that it provokes strokes5.
Quitting smoking can be a particular challenge. According to a study, over 30 attempts to quit may be necessary to quit smoking6. Nicotine dependence is a complex disorder. In fact, it has been observed that the greater the nicotine dependence, the lower the probability of success7, motivation being one of the main factors to quit the habit8. As a consequence, development and optimization of behavioral strategies to quit smoking are gaining relevance.
Behavioral support strategies increase dropout rates9. In the last few years, a wide range of platforms and strategies of behavioral therapy based on clinical-patient health advice have been developed including the telephone line, text messaging, webbased interventions and mobile applications that can be used independently or as an adjuvant tool10. Whittaker et al.11 published in 2016 a meta-analysis of interventions based on mobile telephones to quit smoking that included all the tools described above except mobile applications (apps). Results showed a beneficial effect compared to usual clinical practice (RR=1.67; 95% CI: 1.46–1.90)11. Other research studies have found similar results12-14. On the other hand, applications to quit smoking have also been developed through mobile phones, though data on this matter are still limited15. In fact, data on the quality of content and the effectiveness of the approach are still scarce16-18. For instance, in a recent systematic review, although authors identified almost 50 mobile applications for smoking cessation, only 4% had suitable scientific support19.
Since the launch of mobile networks in the 1980s, the use of mobile phones has grown exponentially. The International Communications Union estimated that by the end of 2015 there would be about 7 billion mobile phones worldwide, that is a penetration of 97%, making these devices increasingly useful for healthcare20. Indeed, numerous applications are now available to provide help with many important tasks including information and time management, maintenance and access to sanitary records, communications and consulting, reference and information gathering, patient management and monitoring, clinical decision making and medical education and training21-23.
The use of health applications among mobile phone users has also increased significantly. In a survey conducted in the United States, it was concluded that a little over half (58.23%) of mobile phone users had downloaded a health-related mobile app. These results were dependent on several factors such as age and educational level, with the age of app users being lower (OR=0.97; 95% CI: 0.96–0.98) than that of non-users, and the higher educational level related to higher app usage (OR=1.12; 95% CI: 1.01–1.24)24. However, in another study evaluating the characteristics of users of mobile applications to quit smoking, it was found that demographic characteristics such as age and education level were not associated with the attitude towards the use of a tobacco cessation application, but rather depended on characteristics related to the content of the application instead of the general characteristics of the users25.
Assuming the wide penetration of mobile telephones and the use of health applications by the population (3.2 billion downloads in 2016)26, we decided to explore whether the use of smoking cessation applications is supported by scientific evidence. To address this issue, a systematic review and meta-analysis of randomized clinical trials evaluating interventions based on mobile applications to quit smoking was carried out.
METHODS
We conducted a systematic review and meta-analysis of clinical trials evaluating the effectiveness of applications for smartphones to quit smoking against other types of therapy that were not a smaller version of the same application, or other different applications (SMS, computer or tablet websites, clinical practice, health advice on smoking). For this purpose, we performed, in December 2019, first a bibliographic search, in MEDLINE, EMBASE and COCHRANE LIBRARY databases with the following search string: (‘Mobile Applications’ [Mesh]) AND (‘Smoking Cessation’ [Mesh]), filtering by type of study including Clinical Trial, Meta-Analysis, Randomized Controlled Trial, and Systematic Review. No language restriction was applied. Given the lack of studies, we also searched in google and other web browsers, and searched for unpublished studies in the platform clinicaltrials.gov.
The studies could be masked and not masked. The main variable evaluated was smoking abstinence. The follow-up period was between 3 and 6 months.
A qualitative and quantitative analysis was performed with selected studies. In the qualitative analysis, risk of bias assessment was carried out (random sequence generation of allocation, concealment of allocation, blinding of participants and staff, blinding of outcome assessors, incomplete outcome data, and selective reporting of results). In the quantitative analysis the risk of publication bias, heterogeneity test, estimation of pooled measure and a sensitivity analysis were performed.
Quality assessment of clinical trials
An evaluation of the risk of bias of the different studies included was carried out using the tool developed by the Cochrane group, which is a domain-based evaluation (random sequence generation of allocation, concealment of allocation, blinding of participants and staff, blinding of outcome assessors, incomplete outcome data, selective reporting of results, other biases)27. Each domain is classified as high, medium or low risk of bias. All studies were included, regardless of the risk of each27.
Heterogeneity analysis
A heterogeneity analysis was performed to evaluate the variability among the studies included in the meta-analysis. To address this, the statistical test Q of DerSimonian and Laird28 was used. Due to low statistical power, the confidence level was set at 90%29. The Q test was completed with the graphics of Galbraith30 and L’Abbé et al.31. The I2 index was also calculated from the equation:
where Q is the Cochran homogeneity test statistic and df is the degrees of freedom (number of trials minus 1). The heterogeneity was considered important if I2 >50%32.
Publication bias
Possible publication bias was assessed using the Egger and Begg statistic and two graphs (Funnel plot and Egger) were constructed. The confidence level for both tests was set at 95%.
Pooled measured estimation
Tobacco cessation was measured by the variable 30 days (30PP) or 7 days point prevalence (7PP) at 6 months from the beginning. To obtain the combined effect for all the studies included in the meta-analysis, the relative risk (RR) and the 95% confidence interval were used.
Sensitivity analysis
The influence of each of the studies on the overall estimation of the effect and, therefore, the robustness of the final measurement, was studied. To address this, the meta-analysis was repeated as many times as the number of the selected studies, skipping one of the studies each time while combining the remaining ones.
RESULTS
A total of nine studies were identified for this meta-analysis. After a more thorough review of the identified studies by the research team, five were discarded because they did not meet the eligibility criteria. In fact, four of them compared different versions of the same application (one more extensive version and another much smaller), and 1 was not a clinical trial but a cross-sectional study evaluating the characteristics of users of web-based programs or websites for mobiles (Figure 1). The characteristics of the four studies33-36 selected are summarized in Table 1. Qualitative analysis was performed with four studies, but the quantitative analysis was only carried out with the studies of Buller et al.35, Danaher al.34, and Baskerville et al.36, given the impossibility of calculating the RR for the study by Peiris et al.33.
Table 1
Studies reviewed
Authors | Title | Sample | Intervention and comparator | Main variables and follow-up | Results |
---|---|---|---|---|---|
Buller et al.35 | Randomized Trial of a Smartphone Mobile Application Compared to Text Messaging to Support Smoking Cessation | Young adult smokers aged 18–30 years (n=102) Of the patients, 58-66% were planning to quit smoking | This study compares a mobile application (REQ-Mobile) (n=51) with text messaging to support smoking cessation (onQ) (n=51) | 12 weeks continuous abstinence (Intention to treat analysis) | RED Q MOBILE 18% (7-28%) ONQ 31% (18-45%) p=0.11 |
Peiris et al.33 | A Smartphone App to Assist Smoking Cessation Among Aboriginal Australians: Findings from a Pilot Randomized Controlled Trial | Current Aboriginal smokers (aged >16 years; mean ± SD 42 ± 14) years), who were willing to make a quit attempt in the next month The majority of patients were smokers of <20 cigarettes/day | The intervention was a multifaceted Android or iOS app comprising a personalized profile and quit plan, text and in-app motivational messages (n=22). The comparator was usual cessation support services (24) | Self-reported continuous smoking abstinence, n (%)at 4 weeks and 6 months visit | Intervention group 0 (0%) Control group 0 (0%) |
Danaher et al.34 | Outcomes and Device Usage for fully automated internet interventions designed for a smartphone or personal computer: The Mobile Quit Smoking cessation randomized controlled trial | 1271 smokers (aged ≥18 years; mean ± SD 42.9 (12.3) years) who wanted to quit in next 14 days Patients were smokers of ≥5 cigarettes/ day in the previous 6 months | Patients were randomly assigned to MobileQuit (n=633) (designed for use in mobile phones) or QuitOnline (n=638) (designed for nonmobile desktop or tablet computers) | Point prevalence self-reported at 3 and 6 months (ITT analysis) | 3 months: MOBILEQUIT: 131 (20.7%) QUITONLINE: 73 (11.4%) p<0.001 6 months: MOBILEQUIT: 156 (24.6%) QUITONLINE: 123 (19.3%) p=0.02 |
Baskerville et al.36 | Effect of a Mobile Phone Intervention on Quitting Smoking in a Young Adult Population of Smokers: Randomized Controlled Trial | 1599 young adult smokers (aged 19–29 years) intending to quit smoking in the next 30 days | Patients were randomized to one of two groups: an evidence-informed smartphone app for smoking cessation, Crush the Crave (CTC) (n=820) and the control group, an evidence-informed self-help guide, On the Road to Quitting (OnRQ) (N=779) | Self-reported continuous abstinence at 6 months follow-up (intention to treat analysis) | CTC: 50 (6.1%) OnRQ: 60 (7.7%) p=0.28 |
Figure 1
PRISMA flow diagram
From: Moher D, Liberati A, Tetzlaff J, Altman DG, The PRISMA Group. Preferred reporting items for systematic reviews and meta-analyses: The PRISMA statement. PLoS Med. 2009;6(6):e1000097. doi:10.1371/journal.pmed.1000097. Prisma statement check list included in Supplementary file.
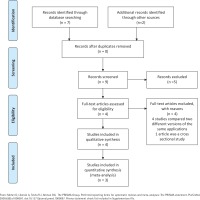
Risk assessment of bias
The risk assessment of bias of each study is analyzed in Figure 2. The main characteristics of each study are now described.
In the study of Peiris et al.33, 49 current Aboriginal smokers (aged >16 years), who were willing to make a quit attempt in the next month, and with access to an iPhone or Android smartphone participated in the clinical trial. The intervention consisted of a personalized profile and quit plan, text and in-app motivational message. The comparator was usual cessation support services. Randomization was conducted through a central computer-based randomization service; therefore, we could think that the randomization sequence was kept hidden until the moment of the assignment. Outcome analysis and data collection were conducted blinded to treatment allocation, but authors do not mention anything about patients. The primary outcome was self-reported continuous smoking abstinence verified by carbon monoxide breath testing at 6 months. Statistical analysis was not carried out by intention to treat. Three people from intervention group were lost in the follow-up.
In the study of Danaher et al.34, 1271 smokers (aged ≥18 years) who wanted to quit were randomly assigned to: 1) MobileQuit (designed for its use in mobile devices), or 2) QuitOnline (designed for non-mobile desktop or tablet computers). Inclusion criteria were smokers who consumed ≥5 cigarettes/day in the previous 6 months and those who wanted to quit smoking in next 14 days.
Participants completed an internet-based registration procedure before being assigned to a condition, via a computer-generated randomization. We could expect that the sequence of concealment was kept hidden until assignment moment. Authors do not mention anything about blinding of participants, researchers, and person responsible for statistical analysis. Statistical analysis was carried out both by intention to treat (participants who did not complete their assessments were considered to be smokers) and by complete cases. The primary variable was 7 days point prevalence at follow-up at 3 and 6 months. The protocol was published in clinicaltrials.org with number NCT01952236.
The study of Buller et al.35 was a clinical trial that included 102 adult smokers aged 18–30 years. Half were assigned to REQMobile, and the other to onQ, using an online randomization program. Patients were registered into the study web site without revealing them to the investigators, but authors do not mention anything about patients. The effect of treatment was analyzed by intention to treat analysis. Patients lost to follow-up were considered as smokers for statistical analysis. In addition, the protocol of this clinical trial was not found. All smokers were invited by e-mail to complete follow-up questionnaires online at 6 and 12 weeks to collect self-reported smoking status. Quitting tobacco was measured at 6 and 12 weeks as 30-day point-prevalence (30 PP), defined as not smoking in the past 30 days.
In the study of Baskerville et al.36, 1520 participants aged 19–29 years were eligible if they were considering quitting smoking in the next 30 days. Those referred to the study by a friend or a family member already participating in the trial, were excluded to avoid possible contamination bias. Patients that met inclusion criteria were randomly allocated to either the control or intervention arm, receiving a computer-generated email confirming registration. Researchers, data collectors, and participants, were blinded to the group assignments. The protocol was available before the study started, as it was registered in clinicaltrials.org with number CT01983150. The statistical analysis was carried out by intention to treat. Follow-up was conducted at 3 and 6 months, post-randomization. Quitting tobacco was measured with the self-reported 30-day point-prevalence abstinence (PPA) from smoking at 3 and 6 months.
Heterogeneity analysis
According to the results of the DerSimonian and Laird11 test, there is statistical heterogeneity among the studies included in the meta-analysis (P<0.05). Similar results can be drawn from the graphs of Galbraith (Figure 3) and L’Abbé (Figure 4). In the former, we can observe that 2 studies are outside the confidence bands, while in the latter, we observe that points were not over a straight line. Despite the heterogeneity between studies, all were maintained in the analysis. I2 index was 76.41%.
Publication bias
The p-value of the statistical tests performed was greater than 0.05, suggesting a lack of publication bias. When analyzing the Funnel Plot and Egger graphs (Supplementary file, Figures S1 and S2), we realized that results do not follow the statistical tests. Thus, the lack of bias cannot be assumed.
Estimation of pooled measurement
The results of the studies included in the meta-analysis have been combined using a random effects model given the existence of heterogeneity. After combining the results, a total RR of 0.901 (95% CI: 0.57-1.423) was estimated (Table 2).
Sensitivity analysis
The study with the greatest influence on the meta-analysis was that of Danaher et al.34. When this study was deleted, and the meta-analysis was repeated, the greatest variation in the estimates of the overall effect was obtained. The estimated RR decreased since a study with a beneficial effect of the intervention to be evaluated was suppressed. The sensitivity analysis showed that the measurement is robust as the direction of the effect does not change and the magnitude of the effect does it minimally (Table 3).
DISCUSSION
After combining the results of the studies included in the meta-analysis, no statistically significant differences are observed between the effectiveness of the app as a tool to quit smoking and the rest of the therapeutic options evaluated. Relative risk for smoking cessation using a mobile application for smartphones versus other type of interventions was 0.901 (95% CI: 0.57-1.423). Studies have been combined using a random effects model given the absence of homogeneity among the included studies.
Strengths and limitations
There are several limitations to the study. First, the heterogeneity is not only statistical but also clinical, since the populations of the included studies are different from each other. Furthermore, the interventions and follow-up periods were not the same for all studies. Indeed, Buller et al.35 did a maximum follow-up of 12 weeks, while in the remaining studies it was 6 months. In relation to the main variable, Danaher et al.34 measured the 7 days point prevalence whereas the remaining studies35,36 evaluated the 30 days point-prevalence abstinence. However, this does not affect our results, because those who have smoked in the past 30 days have also smoked in the past 7 days.
Despite the limitations, the present meta-analysis study has several important strengths. First, an analysis of the risk of bias of the four studies was carried out, showing that the risk of bias in most domains is not high. Second, an analysis of publication bias was performed, detecting that there is, indeed, a risk of publication bias, due to the recent boom of smartphone applications. Accordingly, many ongoing studies will provide results and conclusions within 2–3 years37-40 and a new meta-analysis study will be welcome. Third, the sensitivity analysis showed the robustness of the measurement with the available data, with no significant changes in the magnitude of the combined measurement when repeating the meta-analysis by deleting a study each time. Fourth, another meta-analysis evaluating the effectiveness of the use of a mobile application compared to other alternatives has not been found in the literature. The only exception is the study of Wittaker et al.41. However, these authors performed different meta-analysis depending on the type of intervention while our objective was to compare the effectiveness of the use of a mobile application against any existing intervention to quit smoking.
Future directions
The internet has revolutionized the computer and communications world. Indeed, new forms of technology have been generated in almost all aspects of life42. New mobile devices have appeared on the market. As a consequence, the number of downloads increased in parallel with the number of mobile phone users. For example, in Spain, it is estimated that 3.8 million applications are downloaded every day. In fact, each smartphone has an average of 39 applications43.
We are facing a global new revolution in medicine. Almost 90% of the world’s population could benefit from the opportunities that mobile technologies represent at a relatively low cost44. A total of 36% of all smartphone owners have mHealth apps on their devices. Interestingly, among those using apps, 60% use mHealth apps in achieving health behavior goals45.
CONCLUSIONS
According to the results presented, we are not able to claim that apps are effective for quitting tobacco. As the boom in mobile applications has been very recent, there are very few clinical trials that evaluate their effectiveness against other types of smoking cessation interventions. Even though some studies with protocols of clinical trials have been published to evaluate the effectiveness of mobile applications compared to other alternatives36-39, the trials are still in progress, and therefore the results have not been included in the meta-analysis. In addition, as the adherence to smoking cessation treatment guidelines is generally low, this may potentially have a negative impact on the effectiveness of apps46. On the other hand, and focusing on the usefulness of health app use, there are several patient characteristics that make the difference. Age and education level are significantly related to mobile health app use. The young and more highly educated are more likely to use them. Although gender is not associated with general mobile health app use, men are more likely to use fitness apps than women, and women are more likely to use nutrition, self-care, and reproductive health apps47. Last, but not least, as it is well known that changes promoted by health advice do not last over time (no more than six months), it would be convenient to design clinical trials with follow-up periods longer than 6 months, at least one year, to have a more suitable evaluation of the app47. Is investing in promoting the use of mobile applications justified, or do we have to continue using tools that have showed effectiveness until now7. In summary, it is necessary to go deeper into the characteristics of each app and into the features of potential users in order to try to maximize adherence and success.